In the vast realm of online shopping, product recommendations have become the guiding compass for consumers navigating an abundance of choices. From suggesting the perfect pair of shoes to predicting a preferred lipstick shade, e-commerce sites leverage sophisticated algorithms to offer personalized product recommendations. These recommendations, often appearing as “You Might Also Like” or “Frequently Bought Together,” are far from random; they’re the result of intricate data and behavior analytics and Artificial Intelligence (AI)-driven methodologies.
Why Product Recommendations Are Important for E-Commerce
Product recommendations play a pivotal role in the success of e-commerce for several reasons. Firstly, they significantly enhance the user experience by personalizing and streamlining the shopping journey. Presenting shoppers with personalized product recommendations makes it easier to discover products that align with their interests, thereby increasing the likelihood of purchase.
Moreover, these suggestions boost engagement and retention by keeping users on the platform longer, offering them a more satisfying and efficient shopping experience. By showcasing relevant items, cross-selling or upselling complementary products, and adapting to changing user preferences, product recommendations increase the chances of multiple purchases and higher average order values.
Additionally, product recommendations serve as a powerful marketing tool. They contribute to increased sales and revenue by strategically highlighting products, promoting new offerings, and effectively targeting customers with relevant promotions or discounts. This targeted approach can improve conversion rates and strengthen customer loyalty and satisfaction.
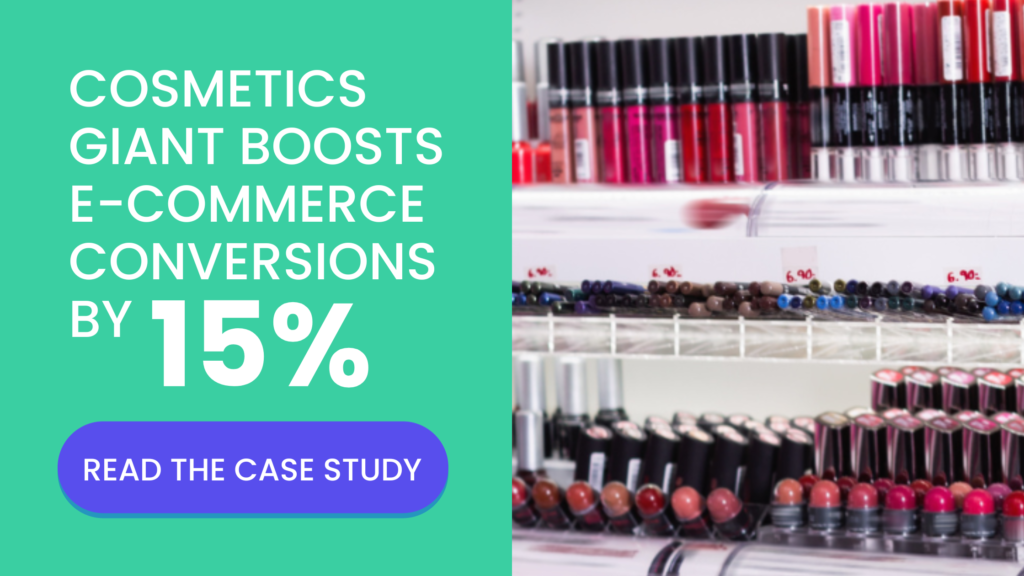
3 Methods to Creating a Personalized Shopping Experience with Recommendations
In personalized product recommendations, three distinct methods drive tailored suggestions for users: data-driven, contextual, and behavioral approaches. These methodologies harness diverse sources of information to curate suggestions aligned with individual preferences and needs.
1. Data-Driven
Personalized product recommendations often use individual user data like known preferences, past interactions, and demographic information, to curate tailored suggestions. Through machine learning and algorithms, websites can analyze this wealth of data to predict and offer products or content that align closely with each user’s unique interests and needs. These recommendations aim to enhance user engagement and satisfaction by presenting a personalized selection that resonates with their specific tastes and behaviors, creating a more enjoyable and efficient user experience.
2. Contextual
Contextual product recommendations leverage user demographics, seasonal trends, real-time data, social influence, and cross-selling opportunities to suggest personalized items. By analyzing this diverse set of data, algorithms generate recommendations that align with a user’s interests, potentially predicting what products they might find appealing. Whether influenced by weather conditions, time of day, or recent or upcoming events, these recommendations aim to enhance the user experience by offering relevant and timely suggestions, ultimately increasing the likelihood of a successful purchase or engagement.
3. Behavioral
Behavioral recommendations come from analyzing a user’s past actions, such as purchases, clicks, searches, and time spent looking at specific items or categories. By scrutinizing these behavioral patterns, algorithms identify trends and preferences to suggest products or content closely aligned with a user’s demonstrated interests and habits. These recommendations leverage the user’s history of interactions to anticipate their future needs, aiming to provide timely and relevant suggestions that cater to their evolving preferences.
Understanding the Mechanisms Behind Personalized Product Recommendations
User Data Collection
The journey starts with data collection. E-commerce platforms gather visitor information, including browsing history, purchase patterns, items added to carts, and demographic details like age, location, and interests. This data has served as the cornerstone for crafting tailored recommendations.
Algorithmic Science
Armed with all of this data, machine learning algorithms step in. These algorithms employ various techniques, such as collaborative filtering, content-based filtering, and hybrid approaches, to analyze and draw correlations between user behavior and product attributes. Collaborative filtering, for instance, examines user preferences by comparing them with others who share similar tastes, while content-based filtering matches product attributes to user preferences.
Constant Learning
The beauty of personalized product recommendations lies in continuous learning. Users’ behavior shapes and refine the algorithms as they interact with the site. This feedback loop ensures that suggestions become increasingly accurate over time.
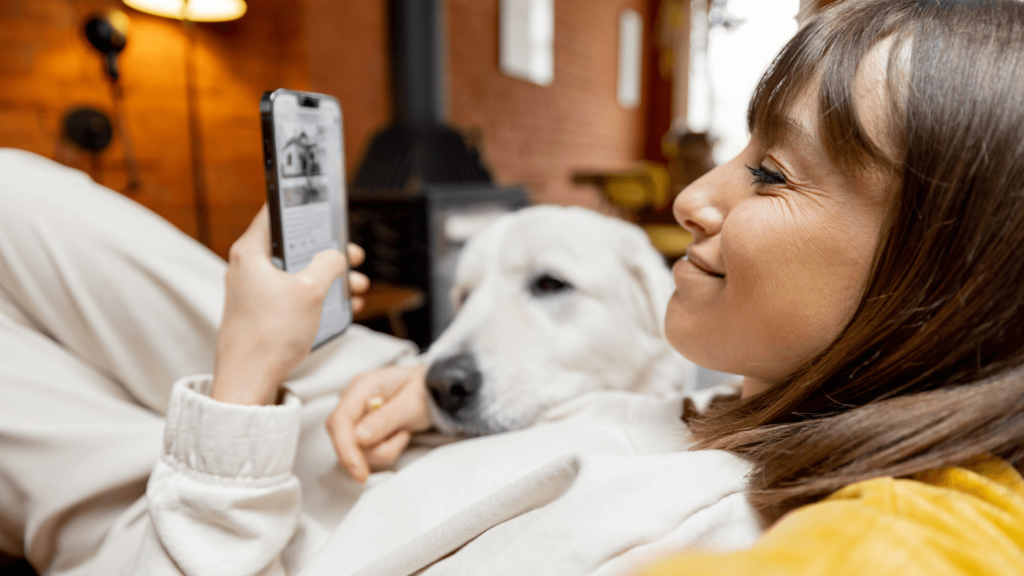
Concerns Around User Data Collection
The power of tailored suggestions, shaped by user behavior and preferences, often raises privacy and data security issues. Striking a balance between personalization and safeguarding user data remains a critical challenge in ensuring a positive user experience while addressing ethical implications associated with recommendation systems.
Privacy concerns surrounding personalized product recommendations stem from the extensive collection, analysis, and storage of user data, specifically information tracked by third-party cookies. Tailoring suggestions has historically relied heavily on accessing and interpreting personal information, including internet browsing history and demographic details gathered by tracking cookies. Balancing the need for personalized shopping experiences with safeguarding user privacy necessitates looking for solutions outside of third-party cookies.
Using AI-Driven Product Recommendation Solutions
With laws and initiatives like GDPR and CCPA, and major browsers phasing out third-party cookies, AI-driven solutions like YouneeqAI have become more prominent in providing personalized product recommendations. These AI algorithms, often based on machine learning models, utilize first-party data directly collected from user interactions on an e-commerce site. This shift enables businesses to maintain personalized experiences while prioritizing user privacy, as AI algorithms work within the confines of a website’s data rather than tracking users across the web. By adapting and learning from ongoing user behavior, AI-driven recommendations continuously refine themselves, striving to offer increasingly accurate and valuable suggestions, ultimately enhancing the overall user experience.
YouneeqAI seamlessly integrates with any website via JavaScript, enabling store owners to quickly onboard and begin the algorithmic learning period. There is no difficult set up or need for an additional platform. Without relying on third-party cookies, intricate user journeys, or CRM data, YouneeqAI ensures every user’s seamless and privacy-focused experience while increasing conversion rates and revenue.
Personalized Product Recommendations for Your Store
Product recommendations are an important aspect of personalized online shopping, significantly impacting conversion rates and e-commerce revenue. By leveraging available user data, paired with sophisticated AI algorithms, e-commerce platforms can provide a customized shopping experience that increases time spent on site, average order value, and customer trust and satisfaction. The future promises an even more intuitive and precise system for personalized product recommendations, fundamentally reshaping the online shopping experience and fueling revenue growth for businesses. To learn more about how AI can help you personalize product recommendations, chat with one of our experts.